The Next Big Tech Gig Will Be the AI Wrangler
Get ready to referee some bot fights.
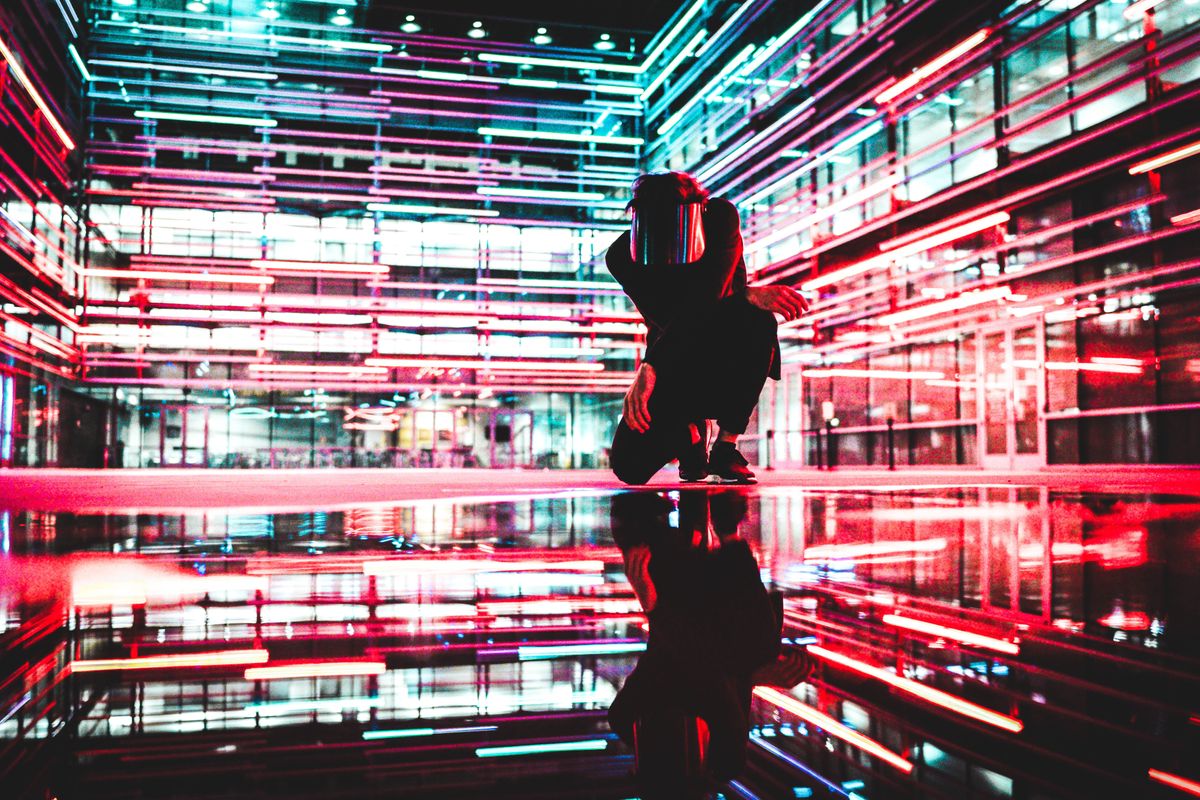
Photo by Drew Graham on Unsplash
If you're in any type of corporate technology gig and are not currently wracking your brain as to whether AI can be integrated into your business, you will be. AI is effectively everywhere as capital searches for ways to apply the technology, and the growth in new companies means that AI is being sold in increasingly creative ways as armies of salesmen backed by investors square off to hammer hundreds of square pegs into round holes.
One out of every five pitches will land, and someone will be given the job to pay attention to the thing leadership bought and approved for integration into your stack. And while in few years the glut of available products will solidify into a tangible marketplace, the flexibility and portability of AI means it will continue to be nimbly applied to plenty of sectors, ensuring that learning applications will keep popping up in previously unexpected sectors for many years to come.
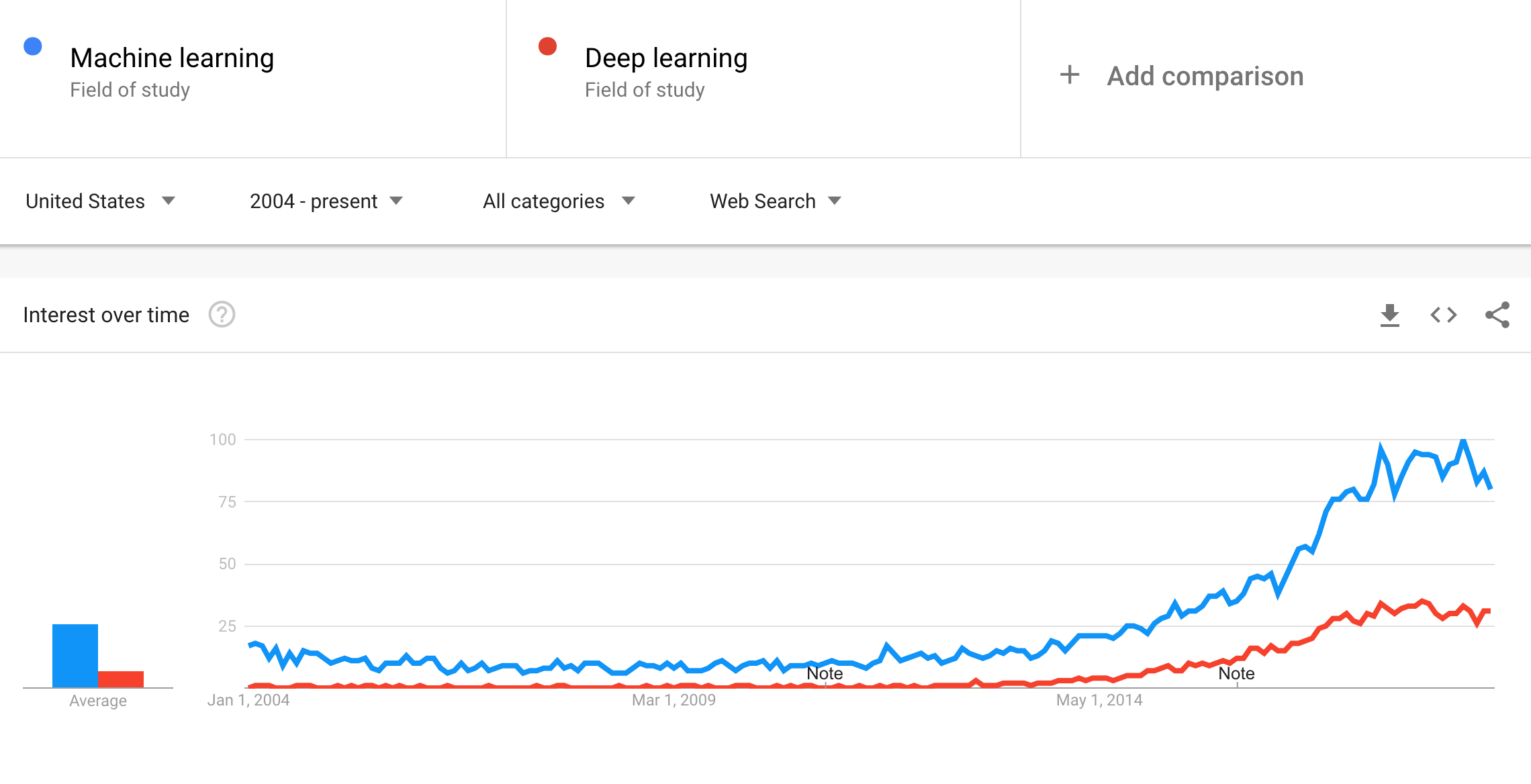
All of this applied AI will need monitoring as it learns, it will need training (particularly at deployment), and (most importantly) it will need to be refereed alongside other new technology.
This world will require new skills and a new type of role. Most importantly, the early leaders in this role will come directly from the very jobs disrupted by AI. As various jobs are reduced or removed, these new roles will arrive to take their place (though probably not in the same volume as before).
This new role is the AI Wrangler. Here’s what they’ll be doing.
Training
When launching a new AI tool from scratch, most solutions architects will suggest training data to jumpstart the learning process. The AI Wrangler will manage this at launch — running through the legal and security reviews, then packaging up the training data for consumption to train the model, or setting up the protocols that will send data to the AI tool. If they’re not using training data at the outset, they will need to set up the protocols to make sure the correct data is learned.
Conversely, they’ll need to scope out what the AI tool *shouldn’t* handle, which in many cases is just as important as what it should be handling. What shouldn’t it do, and what exceptions should be put in place so that it will never take a specific action? They will then need to support testing the model and running point on whether it’s hitting expected outcomes. It won’t immediately get much right, and the Wrangler will need to provide additional training, time and…
Fine Tuning and QA
The AI enthusiast will tell you that these are set and forget tools — let them run long enough and they’ll eventually become your new best friend. In reality and just like any human being, they require regular check-ins and check-ups. At the macro level of the role, the Wrangler (perhaps the Director of Wranglers) will need to review how AI is clustering answers to questions and generally performing against past and future metrics. At a transactional level, the AI Wrangler (say AI Wrangler Level 1) will be dropping into specific interactions, QAing individual clusters, and generally administering the tool to fine tune the AI. If the answer to a question was mildly incorrect or had some missing data point, the L1 Wrangler will adjust the AI at the point of interaction. Whole cadres of L1 Wranglers will sprinkle learning moments across tens of thousands of individual AI actions.
Is this simply a transitory period while the Wrangler works themselves out of a job? Unlikely. As new trends emerge, incidents occur, and otherwise unexpected data arrives, Wranglers will be necessary to QA the AI’s reaction to these trends on a granular and macro level. Fine tuning and QA for the best possible experience never stops.
Refereeing the Bot Fights
The “fun” part of Wrangling will be in figuring out how to referee bot fights. In practice, AI tools are built in vacuums. Every tool is created to provide a discrete solution to a discrete problem, and not to interact with or consider other AI. They’re built for ecosystems and to run on systems whose logic is understandable. Other separately running AI is inherently disruptive, not least because they can potentially create feedback loops, red herring results, and otherwise run counter to the objectives of other AI.
There will likely be several running within a system at any given moment, and all will have been built to varying standards by different entities. What's more - these tools are essentially black boxes: algorithmic secret sauces that very few save the original creators understand.
It will be the Wranglers job to arrive at a solution to problems created by AI without necessarily knowing how it functions. They will set up rules (and monitor those rules) to mediate the interactions of these bots.
For example: when Bot A conducts an action, it is triggering Bot B to take another action 15% of the time, introducing junk data into department reporting. The Director of Wrangling and her VP of Engineering are angry that reporting is being disrupting with this incorrect data. The Wrangler is going to have to find a way to mitigate this issue without losing the business value of either tool.
The term "refereeing" in this case is very apt. Solutions to bot fights won't come from within the tool but in designing systems and environments around the bot so that it reacts in a way that is either supplemental to other bots around it, or constrained and cordoned off so as to create less disruption overall. For AI working on similar problems, solutions will need to be mediated and a "victor" chosen.
Preparing for This Future
Initially, the best Wranglers will be those that understand the intended outcomes of AI the best. They will come from the very fields that AI is set to disrupt. Ahead of this transition, future Wranglers should learn the basics of how AI functions — in particular how it learns and categorizes results, and what those statistical frameworks look like.
Where possible they should get real-world experience managing and working with AI functions. More than likely AI is already running on a core system important to their business, which means there’s an opportunity for someone to step up and take point on administering, QAing, and training it.
Finally: they will be prepared to embrace change. AI is simply one more technology in a long line of disruptive advances that work to efficiently reduce labor costs. Until we as a society catch up and figure out the macro problem of sustaining everyone in a low effort environment, it will be necessary to engage with and work with this technology as it impacts our businesses.